10.1371/journal.pone.0154446
http://scihub22266oqcxt.onion/10.1371/journal.pone.0154446
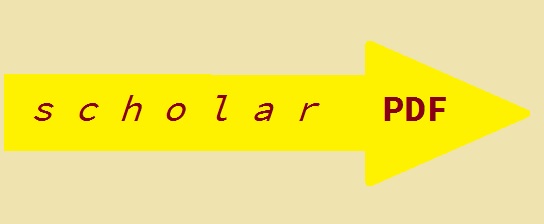 C4849582!4849582!27124604
free
free
free
|  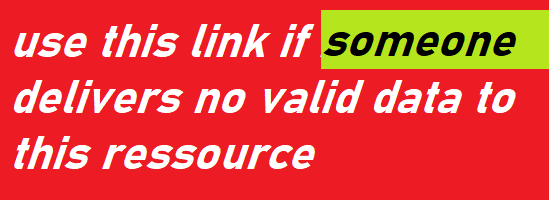
Deprecated: Implicit conversion from float 219.6 to int loses precision in C:\Inetpub\vhosts\kidney.de\httpdocs\pget.php on line 534
Deprecated: Implicit conversion from float 219.6 to int loses precision in C:\Inetpub\vhosts\kidney.de\httpdocs\pget.php on line 534
PLoS+One 2016 ; 11 (4): ä Nephropedia Template TP
gab.com Text
Twit Text FOAVip
Twit Text #
English Wikipedia
|