http://scihub22266oqcxt.onion/
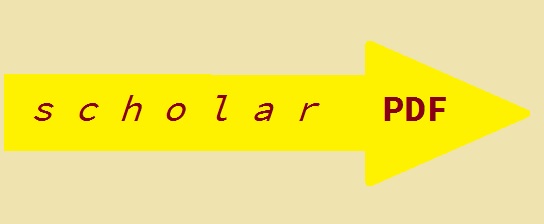 C4803641!ä!Cä
free
free
free
Warning: file_get_contents(https://eutils.ncbi.nlm.nih.gov/entrez/eutils/elink.fcgi?dbfrom=pubmed&id=C�&cmd=llinks): Failed to open stream: HTTP request failed! HTTP/1.1 429 Too Many Requests
in C:\Inetpub\vhosts\kidney.de\httpdocs\pget.php on line 215
|  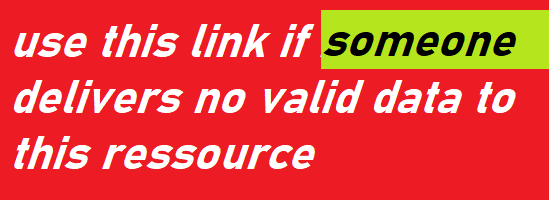
Warning: imagejpeg(C:\Inetpub\vhosts\kidney.de\httpdocs\phplern\C�.jpg): Failed to open stream: No such file or directory in C:\Inetpub\vhosts\kidney.de\httpdocs\pget.php on line 117
ä-/-ä ä ; ä (ä): ä Nephropedia Template TP
gab.com Text
Twit Text FOAVip
Twit Text #
English Wikipedia
|