10.1007/s10278-014-9705-0
http://scihub22266oqcxt.onion/10.1007/s10278-014-9705-0
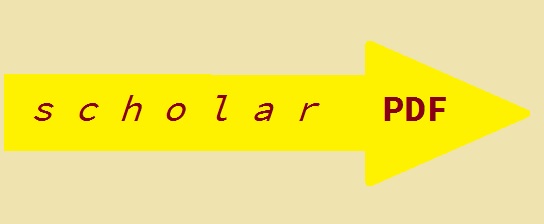 C4391067!4391067!24895064
free
free
free
|  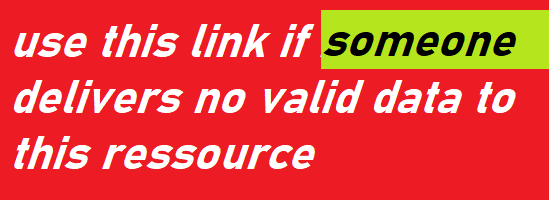
Deprecated: Implicit conversion from float 211.6 to int loses precision in C:\Inetpub\vhosts\kidney.de\httpdocs\pget.php on line 534
Deprecated: Implicit conversion from float 211.6 to int loses precision in C:\Inetpub\vhosts\kidney.de\httpdocs\pget.php on line 534
Deprecated: Implicit conversion from float 211.6 to int loses precision in C:\Inetpub\vhosts\kidney.de\httpdocs\pget.php on line 534
Deprecated: Implicit conversion from float 211.6 to int loses precision in C:\Inetpub\vhosts\kidney.de\httpdocs\pget.php on line 534
J+Digit+Imaging 2014 ; 27 (6): 794-804 Nephropedia Template TP
gab.com Text
Twit Text FOAVip
Twit Text #
English Wikipedia
|