10.1177/11779322211030364
http://scihub22266oqcxt.onion/10.1177/11779322211030364
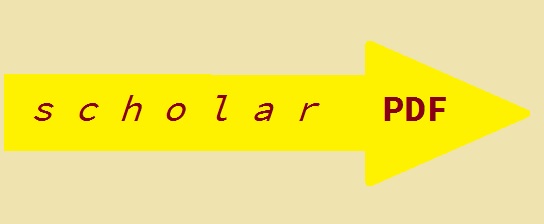 34290496!8274096!34290496
free
free
free
|  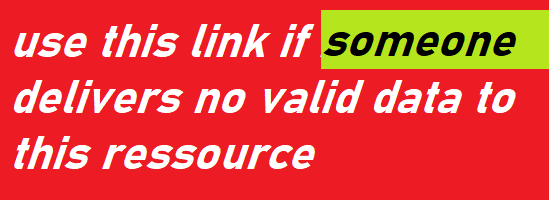
Deprecated: Implicit conversion from float 211.6 to int loses precision in C:\Inetpub\vhosts\kidney.de\httpdocs\pget.php on line 534
Deprecated: Implicit conversion from float 211.6 to int loses precision in C:\Inetpub\vhosts\kidney.de\httpdocs\pget.php on line 534
Deprecated: Implicit conversion from float 211.6 to int loses precision in C:\Inetpub\vhosts\kidney.de\httpdocs\pget.php on line 534
Bioinform+Biol+Insights 2021 ; 15 (ä): 11779322211030364 Nephropedia Template TP
gab.com Text
Twit Text FOAVip
Twit Text #
English Wikipedia
|